Computer Science/Optimization
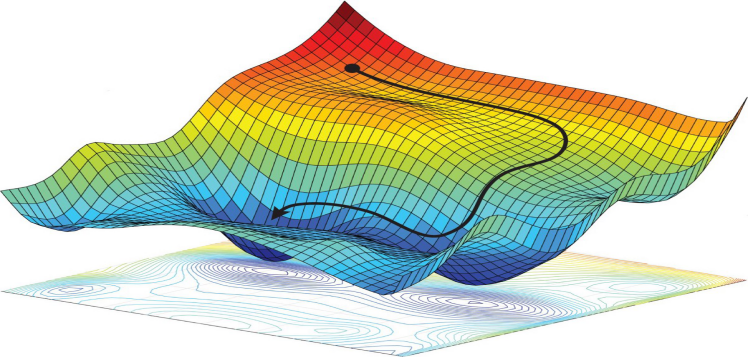
-
Norm approximationBasic norm approximation problemAssume that the columns of AAA are independent.minimize ∥Ax−b∥\text{minimize }\|Ax - b\| minimize ∥Ax−b∥where A∈Rm×nA\in \R^{m\times n}A∈Rm×n with m≥nm\ge nm≥n, ∥⋅∥\|\cdot\|∥⋅∥ is a norm of Rm\R^mRmGeometric interpretationGeometrically, the solution x∗x^*x∗ is the point such that Ax∗∈R(A)Ax^*\in \mathcal R(A)Ax∗∈R(A) that closest to bbb. ..
6. Approximation and fittingNorm approximationBasic norm approximation problemAssume that the columns of AAA are independent.minimize ∥Ax−b∥\text{minimize }\|Ax - b\| minimize ∥Ax−b∥where A∈Rm×nA\in \R^{m\times n}A∈Rm×n with m≥nm\ge nm≥n, ∥⋅∥\|\cdot\|∥⋅∥ is a norm of Rm\R^mRmGeometric interpretationGeometrically, the solution x∗x^*x∗ is the point such that Ax∗∈R(A)Ax^*\in \mathcal R(A)Ax∗∈R(A) that closest to bbb. ..
2024.02.23 -
LagrangianThe basic idea in Lagrangian duality is to take the constraints into account by augmenting the objective function with a weighted sum of the constraint functions.💡By using the Lagrangian, we can get a hint to solve the original problem which is not convex nor easy to solve.Standard form problem (not necessary convex)minimize f0(x)\text{minimize }f_0(x)minimize f0(x)subject tofi(x)≤0i=..
5. DualityLagrangianThe basic idea in Lagrangian duality is to take the constraints into account by augmenting the objective function with a weighted sum of the constraint functions.💡By using the Lagrangian, we can get a hint to solve the original problem which is not convex nor easy to solve.Standard form problem (not necessary convex)minimize f0(x)\text{minimize }f_0(x)minimize f0(x)subject tofi(x)≤0i=..
2024.02.13 -
Optimization problem in standard formminimize f0(x)\text{minimize } f_0(x)minimize f0(x)subject tofi(x)≤0, i=1,…,mhi(x)=0, i=1,…,pf_i(x) \le 0, \; i = 1, \dots, m \\ h_i(x) = 0, \; i = 1, \dots, pfi(x)≤0,i=1,…,mhi(x)=0,i=1,…,px∈Rnx\in \R^nx∈Rn is the optimization variablef0:Rn→Rf_0:\R^n\to \Rf0:Rn→R is the objective or cost functionfi:Rn→R, i=1,…,mf_i:\R^n\to \R, \; i = 1, \dots, mfi:R..
4. Convex optimization problemsOptimization problem in standard formminimize f0(x)\text{minimize } f_0(x)minimize f0(x)subject tofi(x)≤0, i=1,…,mhi(x)=0, i=1,…,pf_i(x) \le 0, \; i = 1, \dots, m \\ h_i(x) = 0, \; i = 1, \dots, pfi(x)≤0,i=1,…,mhi(x)=0,i=1,…,px∈Rnx\in \R^nx∈Rn is the optimization variablef0:Rn→Rf_0:\R^n\to \Rf0:Rn→R is the objective or cost functionfi:Rn→R, i=1,…,mf_i:\R^n\to \R, \; i = 1, \dots, mfi:R..
2024.02.06 -
Convex functionf:Rn→Rf:\R^n \to \Rf:Rn→R is convex if dom f\text{dom }f dom f is a convex set andf(θx+(1−θ)y)≤θf(x)+(1−θ)f(y)f(\theta x + (1 - \theta)y ) \le \theta f(x) + (1-\theta)f(y)f(θx+(1−θ)y)≤θf(x)+(1−θ)f(y)for all x,y∈dom f,0≤θ≤1x, y\in \text{dom }f, 0 \le\theta \le 1x,y∈dom f,0≤θ≤1fff is strictly convex if dom f\text{dom }fdom f is convex andf(θx+(1−θ)y)θf(x)+(1−θ)f(y)f(\theta x +..
3. Convex functionConvex functionf:Rn→Rf:\R^n \to \Rf:Rn→R is convex if dom f\text{dom }f dom f is a convex set andf(θx+(1−θ)y)≤θf(x)+(1−θ)f(y)f(\theta x + (1 - \theta)y ) \le \theta f(x) + (1-\theta)f(y)f(θx+(1−θ)y)≤θf(x)+(1−θ)f(y)for all x,y∈dom f,0≤θ≤1x, y\in \text{dom }f, 0 \le\theta \le 1x,y∈dom f,0≤θ≤1fff is strictly convex if dom f\text{dom }fdom f is convex andf(θx+(1−θ)y)θf(x)+(1−θ)f(y)f(\theta x +..
2024.02.02 -
Affine setLine : all points through x1,x2x_1, x_2x1,x2x=θx1+(1−θ)x2x = \theta x_1 + (1 - \theta) x_2x=θx1+(1−θ)x2where θ∈R\theta\in \Rθ∈RThis idea can be generalized to more than two points.θ1x1+θ2x2+⋯+θkxk\theta_1x_1 + \theta_2 x_2 + \cdots + \theta_kx_kθ1x1+θ2x2+⋯+θkxkwhere θ1+⋯+θk=1\theta_1 + \cdots + \theta_k = 1θ1+⋯+θk=1We refer to a point can be expressed as the following fo..
2. Convex setAffine setLine : all points through x1,x2x_1, x_2x1,x2x=θx1+(1−θ)x2x = \theta x_1 + (1 - \theta) x_2x=θx1+(1−θ)x2where θ∈R\theta\in \Rθ∈RThis idea can be generalized to more than two points.θ1x1+θ2x2+⋯+θkxk\theta_1x_1 + \theta_2 x_2 + \cdots + \theta_kx_kθ1x1+θ2x2+⋯+θkxkwhere θ1+⋯+θk=1\theta_1 + \cdots + \theta_k = 1θ1+⋯+θk=1We refer to a point can be expressed as the following fo..
2024.01.26 -
Mathematical optimizationminxfo(x)s.t fi(x)≤bi, i=1,…,m\begin{align}\min_x & f_o(x) \\ \text{s.t }& f_i(x) \le b_i, \ i = 1, \dots, m\end{align}xmins.t fo(x)fi(x)≤bi, i=1,…,mwherex=(x1,…,xn)x = (x_1, \dots, x_n)x=(x1,…,xn): optimization variablesfo:Rn→Rf_o : R^n \to Rfo:Rn→R: objective function (a.k.a the function we want to minimize)💡In deep learning or machine learning perspective..
1. IntroductionMathematical optimizationminxfo(x)s.t fi(x)≤bi, i=1,…,m\begin{align}\min_x & f_o(x) \\ \text{s.t }& f_i(x) \le b_i, \ i = 1, \dots, m\end{align}xmins.t fo(x)fi(x)≤bi, i=1,…,mwherex=(x1,…,xn)x = (x_1, \dots, x_n)x=(x1,…,xn): optimization variablesfo:Rn→Rf_o : R^n \to Rfo:Rn→R: objective function (a.k.a the function we want to minimize)💡In deep learning or machine learning perspective..
2024.01.26 -
Summary 2024.01.05
-
IntroductionA baker with two types of cakes: simple and fancyBoth types require some basic ingredients; the fancy type requires fancier ingredients and more labor but generates more profit.Popular bakery: No upper limit on the quantity that can be soldBaker’s problem: How many simple and fancy cakes to produce?max24x1+14x2\max 24x_1 + 14x_2max24x1+14x2subject to\text{subject to}subject to3x1..
14. DualityIntroductionA baker with two types of cakes: simple and fancyBoth types require some basic ingredients; the fancy type requires fancier ingredients and more labor but generates more profit.Popular bakery: No upper limit on the quantity that can be soldBaker’s problem: How many simple and fancy cakes to produce?max24x1+14x2\max 24x_1 + 14x_2max24x1+14x2subject to\text{subject to}subject to3x1..
2023.12.19