7. Support Vector Machine
- -
Functional Margin and Geometric Margin
What is the difference
The key difference between the functional margin and geometric margin is that the functional margin is defined for every data point
, whereas the geometric margin is defined only for the data point closest to the decision boundary
. The geometric margin is often used as a measure of the generalization performance of a linear classifier, as it captures how well the classifier can separate new data points that are similar to the training data.
Functional Margin
Given a training example (x(i),y(i)), we define the functional margin
of (w,b) with respect to the training example
Note that if y(i)=1, then for the functional margin to be large (i.e. for our prediction to be confident and correct), we need wTx+b to be a large positive
number. Conversely, if y(i)=−1, then for the functional margin to be large, we need wTx+b to be a large negative
number.
confident
and a correct predictionGiven a training set S={(x(i),y(i))∣i=1,…,m}, we can define the functional margin of (w,b) with respect to S to be the smallest of the functional margins of the individual training examples. Denoted by r^, this can be written as
However, there’s one property of the functional margin that makes it not a very good measure of confidence. This is because if we replace w with 2w and b with 2b, then since g(wTx+b)=g(2wt+2b), this would not change the result of classifier (Since, classifier and g depends only on the sign
, but not on the magnitude.
This means that we can scale w and b without changing the result of classifier. Intuitively, it might therefore makes sense to impose some sort of normalization condition such as ∥w∥2=1 (i.e. we can replace w and b with w/∥w∥2 and b/∥w∥2 respectively. So the concept of geometric margin arises.
Geometric margin
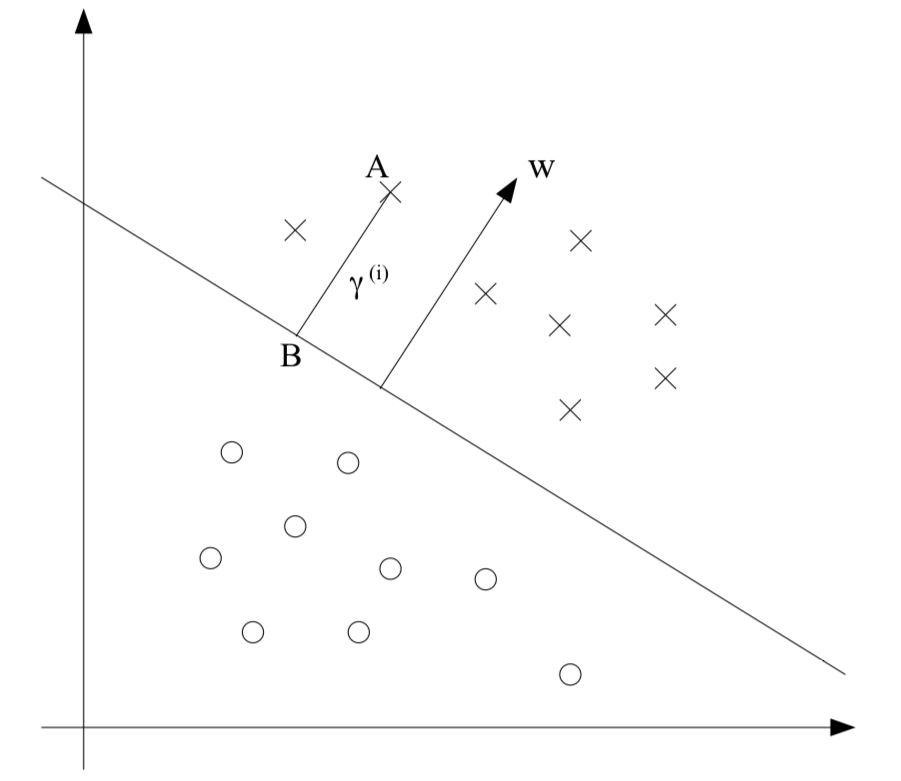
We want to show that geometric margin is not only the normalizing functional margin but also the actual geometric distance between the datapoint and the decision boundary. Since we already know that A represents x(i), we therefore find that the point B is given x(i)−r(i)⋅w/∥w∥. In addition, we already know that the point B lies in the decision boundary. Therefore
Solving for r(i) yields
Therefore, the geometric margin is invariant
to rescaling of the parameters.
In addition, given a training set S={(x(i),y(i))∣i=1,…,m}, we also define the geometric margin of (w,b) with respect to S to be the smallest of the geometric margins on the individual training examples:
The optimal margin classifier
Given a training set, we want to find the optimal decision boundary that maximizes the geometric margin. We will assume that we are given a training set that is linearly separable.
So, we want to solve the following optimization problem
But this optimization problem has “∥w∥=1” constraint which is not a convex
. So, we have to reformulate the above optimization problem as follows
However, the objective function(∥w∥r^) still non-covex function
, so we need to step further. Recall our earlier discussion that we can add an arbitrary scaling constraint on w and b without changing anything
. By using this fact, we want to make the functional margin of w,b with respect to the training set to be 1:
Therefore, the optimization problem is reformulated as follows
However, the objective function(∥w∥1) still non-convex function
, so we need to step further.
It’s easily show that maximizing ∥w∥1 is equivalent to minimizing ∥w∥2 which is convex. Finally, therefore, we can derive the optimization problem as follows
Although the above objective function can be solved by quadratic programming, if we use Lagrange duality
, we can use kernels
to get optimal margin classifiers to work efficiently in very high dimensional space.
Lagrange duality
Consider a problem of the following form:
This problem could be solved by Lagrange multipliers
. In this method, we can define the Lagrangian
to be
Here, the βi’s called the Lagrange multipliers
. We would then find and set L’s partial derivative to zero:
and solve for w and β. The above optimization problem only has equality constraint. What if the optimization problem has inequality constraint such as
To solve it, we start by defining the generalized Lagrangian
.
Consider the quantity
where P means primal
. We can easily find that
Therefore, our original optimization problem can be reformulated as follows
Now, let’s look at a a slightly different problem. Define
where D means dual
. We can define the dual
optimization problem:
the order of min and max
.Normally, d∗≤p∗. However, under certain conditions, we will have
if these conditions hold.
- f and the gi’s are convex, and hi’s are affine.
- The constraints gi are strictly feasible; this means that there exists some w so that gi(w)<0 for all i.
Under above assumptions, there must
exist w∗,α∗,β∗ so that w∗ is the solution to the primal problem. α∗,β∗ are the solutions to the dual problem, and moreover p∗=d∗=L(w∗,α∗,β∗). Moreover, w∗,α∗, and β∗ satisfy the KKT conditions
, which are as follows:
Moreover, if some w∗,α∗,β∗ satisfy the KKT conditions, then it is also a solution to the primal and dual problems.
More specifically, one of the most important condition in KKT conditions is
which is called KKT dual complementarity condition
. This implies that if αi∗>0, then gi(w∗)=0 (i.e. The inequality constraints holds with equality rather than with inequality)
support vectors
Revisit the optimal margin classifier
As I mentioned, the final optimization problem we want to solve is
We can write the constraints as
Therefore, by KKT dual complementary conditions, αi>0 only for the training examples that have functional margin exactly equal to 1.
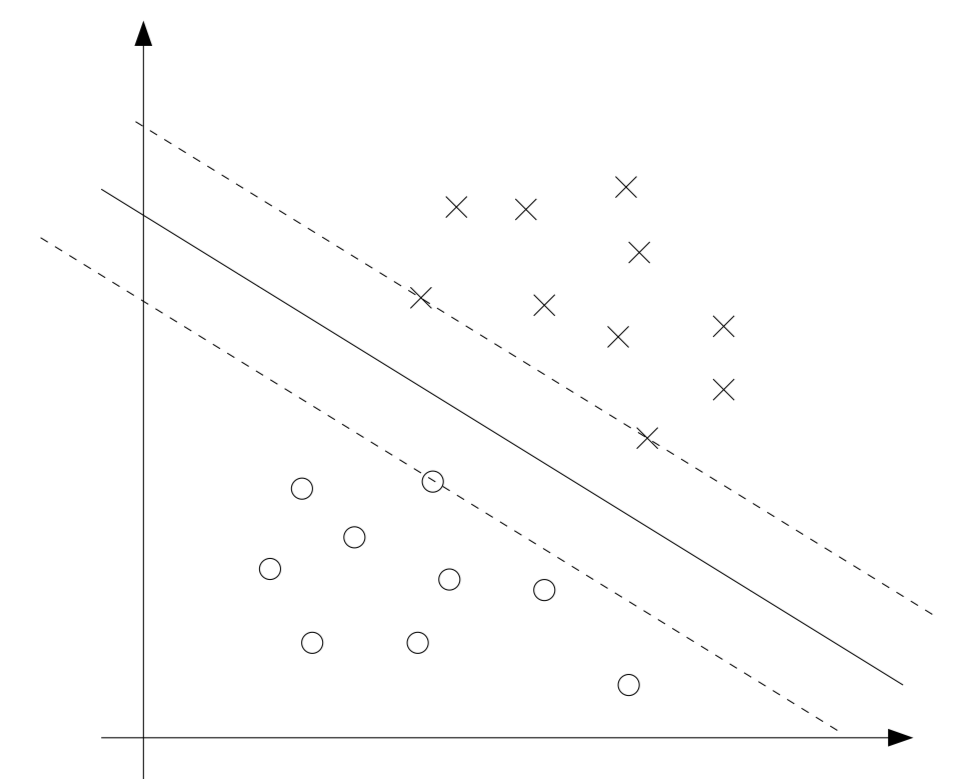
The points with the smallest margins are the exactly the ones closest to the decision boundary. Thus, only three of the αi’s that corresponds to the three training example which has the smallest margins have non-zero at the optimal solution. These three points are called the support veectors
When we construct the Lagrangian for our optimization problem we have:
Let’s find the dual form of the problem. To do so, we need to first minimize L(w,b,α) with respect to w and b, to get θD.
This implies that
By using these equations, we can reformulate the original Lagrangian as follows
Therefore, we obtain the following dual optimization problem
:
After we find α∗, we can easily derive w∗ by using w=∑i=1mαiy(i)x(i). In addition, we can also find b∗ by using following fact
Suppose we’ve fit our model’s parameters to a training set, and now wish to make a prediction at a new point input x. We would then calculate w∗x+b, and predict y=1 iff this quantity is bigger than zero. By using w=∑i=1mαiy(i)x(i), we can derive
Hence, if we’ve found the αi’s, in order to make a prediction, we have to calculate a quantity that depends only on the inner product
between x and the points in the training set. Moreover, we saw earlier that the αi’s will all be zero except for the support vectors
. So we really need to find only the inner products between x and the support vectors
.
Meaning of Basis function
A basis function is a function used to construct a particular function or set of functions as a linear combination of the basis function. Let f is a function which is we wanted to find. We can construct this function as a linear combination of the basis function.
Since span{ϕ1,⋯,ϕL} is isomorphic to RL, we can think
So, we can think that
And we want to take cost function to
when we have n data.
We want to find the optimal θ (i.e. we want to regard this function as a function of θ)
Batch gradient descent
So, gradient descent for θ is following (Batch gradient descent case)
Stochastic gradient descent
For i in range(1, n):
Conclusion
Using basis functions can help increase the dimensionality of the input space, which can make it easier to find decision boundaries or capture more complex relationships between the inputs and outputs.
Above example shows that input space is d-dimensional, however, output space is L-dimensional.
Kernel Trick
Actually, we can express θ as a linear combination of ϕ(x1),⋯,ϕ(xn) which are generated by input values.
When we update the value of θ by using batch gradient descent twice, we can easily find that
Let βi:=βi+α(y(i)−θTϕ(x(i))), we can easily found that θ could be expressed by just linear combination of ϕ(x1),⋯,ϕ(xn).
Since θ could be express by linear combination of ϕ(x1),⋯,ϕ(xn),
So, from now on, we are focusing on finding an optimal value of β.
Why we have to do this procedure
- ⟨ϕ(x(j)),ϕ(x(i))⟩ can be
pre-calculated
for all pairs of i and j.
- In addition, we don’t have to compute ϕ(x(i)) explicitly, when we try to calculate ⟨ϕ(x(j)),ϕ(x(i))⟩
Regularization and the non-separable case
The derivation of the SVM as presented so far assumed that the data is linearly separable.However, we can’t guarantee that it always will be so. In addition, the original SVM approach is very sensitive to outliers.
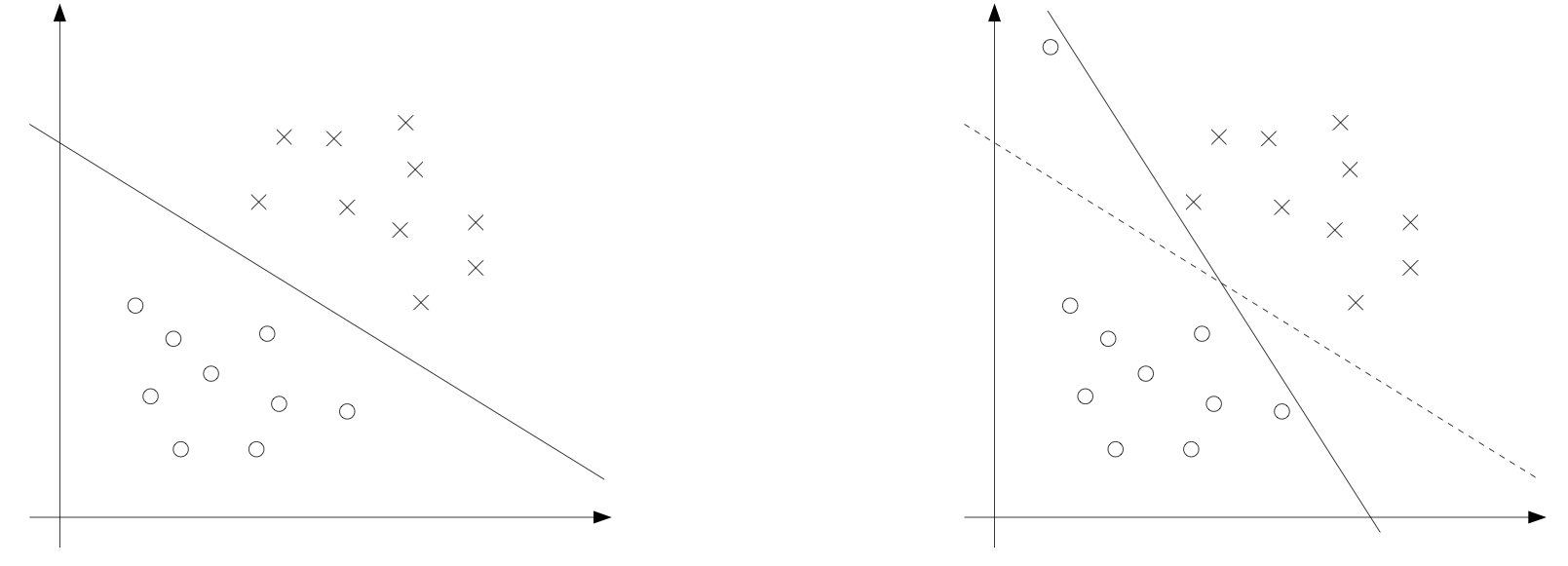
The above figure shows that just one outlier changes the decision boundary dramatically. To make the algorithm work for non-linearly separable datasets as well as be less sensitive to outliers, we reformulate our optimization (using l1 regularization
) as follows
As before, we can form the Lagrangian:
After we calculate the dual form, we can derive
소중한 공감 감사합니다